The Process Improvement Explorer (PIX)
Automated Discovery and Assessment of Business Process Improvement Opportunities
ERC Advanced Grant, 2019-2024
Principal investigator: Marlon Dumas, University of Tartu
Senior Investigators: Fredrik Milani, Alexander Nolte, Orlenys López-Pintado, David Chapela-Campa
Collaborators Wil van der Aalst (RWTH Aachen), Saso Dzeroski (Jozef Stefan Institute), Avigdor Gal (Technion), Marcello La Rosa (University of Melbourne), Jan Mendling (WU Vienna), Hajo A. Reijers (Utrecht University)
Project Overview
Business processes are the operational backbone of modern organizations. Their continuous improvement is key to the achievement of business objectives, be it with respect to efficiency, quality, compliance, or agility. Accordingly, a common task for process analysts is to discover and assess process improvement opportunities, i.e. changes to one or more processes, which are likely to improve them with respect to one or more performance measures.
Current approaches to discover business process improvement opportunities are expert-driven. In these approaches, models and execution data are used to assess opportunities derived from experience and intuition rather than to discover them in the first place. Moreover, as the assessment of opportunities is manual, analysts can only explore a fraction thereof.
PIX will build the foundations of a new generation of process improvement methods that do not exclusively rely on guidelines and heuristics, but rather on a systematic exploration of a space of possible changes derived from process execution data. Specifically, PIX will develop conceptual frameworks and algorithms to analyze process execution data in order to discover possible improvement opportunities. The project will focus on four types of improvement opportunities:
- Task automation: Discovering tasks or sub-tasks that are deterministic and can be partially or fully automated using, for example, Robotic Process Automation (RPA) technology
- Control-flow optimization: Discovering changes in the control-flow of a process (e.g. re-ordering of tasks, task merging/splitting), which can lead to lower cycle times, lower costs, or less SLA violations.
- Decision optimization: Refining the decision logic of the process in order to reduce wastes or reducing defect rates, for example by determining that under certain conditions, executing a given activity leads to lower defect rates than not postponing it or skipping it.
- Resource optimization: Fine-tuning the resource allocation logic in a process or fine-tuning the prioritization of tasks in order to reduce costs or decrease cycles times.
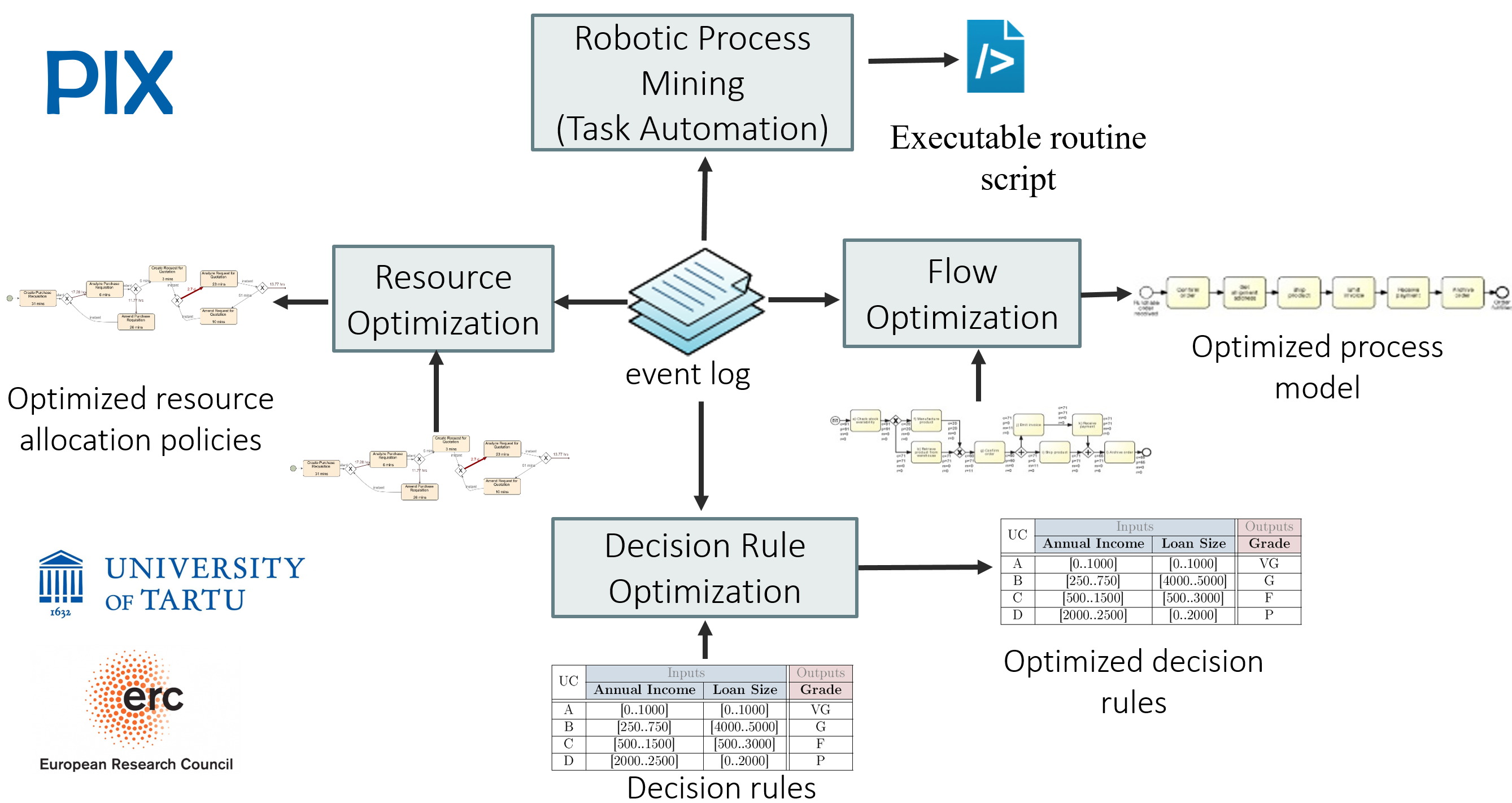
Each change will be associated with a multi-dimensional utility, thus allowing us to map a process improvement problem to an optimization problem over a multidimensional space. Given this mapping, PIX will develop efficient and incremental methods to search through said spaces in order to find Pareto-optimal groups of changes.
The outputs of the project will be embodied in a first-of-its-kind tool for automated process improvement discovery, which will lift the focus in the field of process mining from analyzing as-is processes to designing to-be processes.
Selected research outputs
- Best Paper Award at RCIS'2022: Data-Driven Analysis of Batch Processing Inefficiencies in Business Processes. See also the slide deck of the paper presentation.
- Research article on robotic process mining published in the journal Information Systems: Discovering data transfer routines from user interaction logs.
- Research article on data-driven simulation published in the journal Data & Knowledge Engineering: Discovering business process simulation models in the presence of multitasking and availability constraints
- Keynote talk on data-driven simulation at the SIMULTECH'2021 Conference (Slides)
- Paper on robotic process mining at ICPM'2020: Identifying Candidate Routines for Robotic Process Automation From Unsegmented UI Logs
- Vision paper published in the journal Business and Information Systems Engineering: Robotic Process Mining: Vision and Challenges. See also the companion animation Robotic Process Mining.
- Paper on casual machine learning at ICPM'2020: Process Mining Meets Causal Machine Learning:Discovering Causal Rules from Event Logs. See also this blog post on Causal Process Mining.
- Talk on Process Mining 2.0: The Future of Process Mining at ICPM'2020 (HSPI booth).
- Prototype demonstration at BPM'2020: Robidium: Automated Synthesis of Robotic Process Automation Scripts from UI Logs. (Video)
- Keynote talk on Automated Process Improvement at BPMDS+EMMSAD'2020, June 2020. (Slides)
- Best paper award at the workshop on Intelligent Process Automation (IPA'2020): Automated Discovery of Data Transformations for Robotic Process Automation
- Research article on data-driven simulation published in the journal Decision Support Systems: Automated discovery of business process simulation models from event logs
- Prototype demonstration at BPM'2019: Simod: A Tool for Automated Discovery of Business Process Simulation Models. (Video)
- Prototype demonstration at BPM'2019: Action Logger: Enabling Process Mining for Robotic Process Automation. (Video)